Electronics, Free Full-Text
Por um escritor misterioso
Descrição
In recent years, deep learning has garnered tremendous success in a variety of application domains. This new field of machine learning has been growing rapidly and has been applied to most traditional application domains, as well as some new areas that present more opportunities. Different methods have been proposed based on different categories of learning, including supervised, semi-supervised, and un-supervised learning. Experimental results show state-of-the-art performance using deep learning when compared to traditional machine learning approaches in the fields of image processing, computer vision, speech recognition, machine translation, art, medical imaging, medical information processing, robotics and control, bioinformatics, natural language processing, cybersecurity, and many others. This survey presents a brief survey on the advances that have occurred in the area of Deep Learning (DL), starting with the Deep Neural Network (DNN). The survey goes on to cover Convolutional Neural Network (CNN), Recurrent Neural Network (RNN), including Long Short-Term Memory (LSTM) and Gated Recurrent Units (GRU), Auto-Encoder (AE), Deep Belief Network (DBN), Generative Adversarial Network (GAN), and Deep Reinforcement Learning (DRL). Additionally, we have discussed recent developments, such as advanced variant DL techniques based on these DL approaches. This work considers most of the papers published after 2012 from when the history of deep learning began. Furthermore, DL approaches that have been explored and evaluated in different application domains are also included in this survey. We also included recently developed frameworks, SDKs, and benchmark datasets that are used for implementing and evaluating deep learning approaches. There are some surveys that have been published on DL using neural networks and a survey on Reinforcement Learning (RL). However, those papers have not discussed individual advanced techniques for training large-scale deep learning models and the recently developed method of generative models.
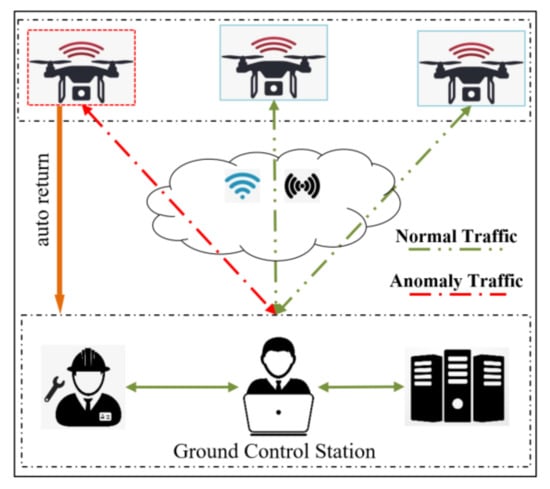
Digital Electronics Circuits And Systems By Puri Free - Colaboratory
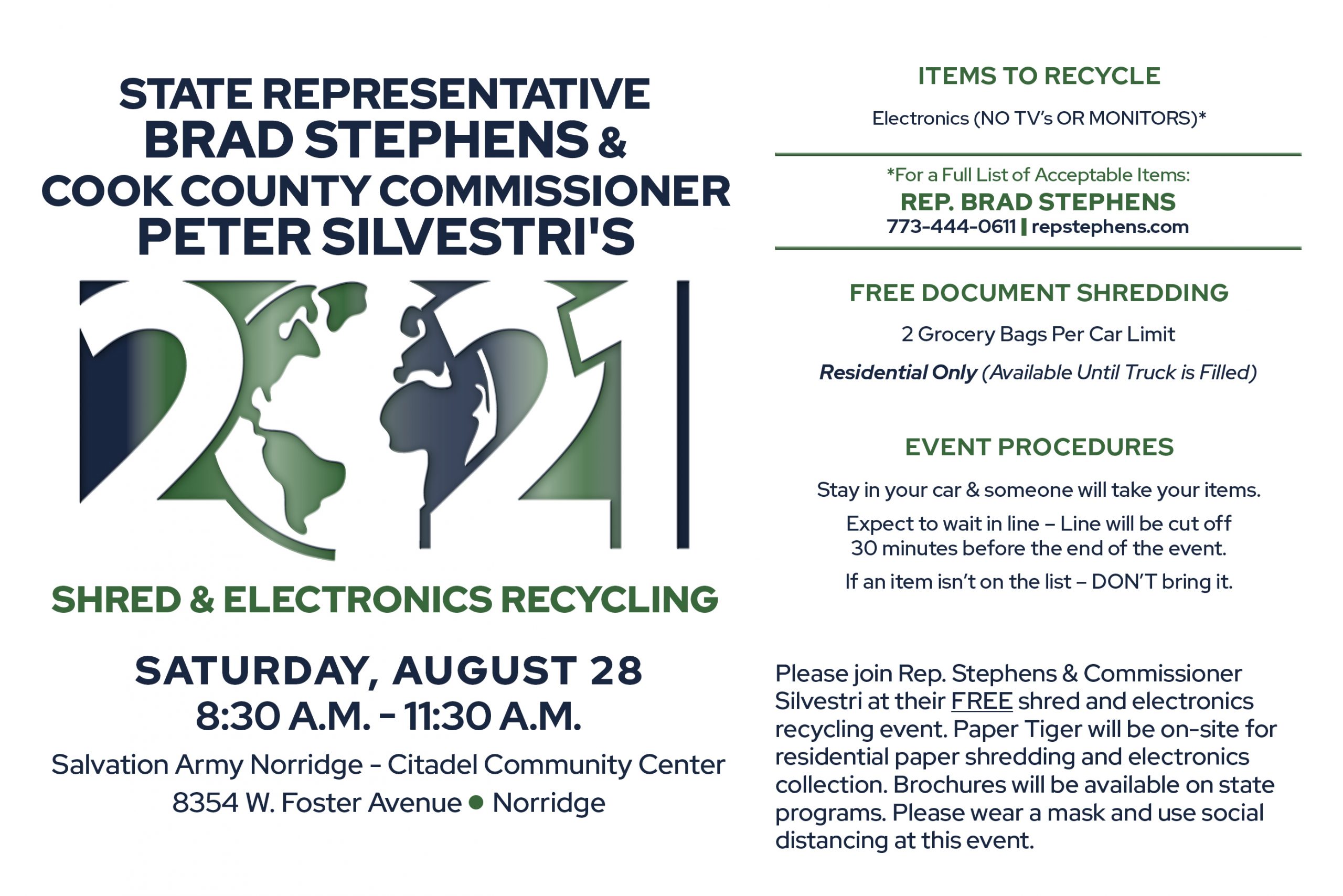
Rep. Stephens & Commissioner Silvestri Host Shred & Electronics Recycling Event - Brad Stephens
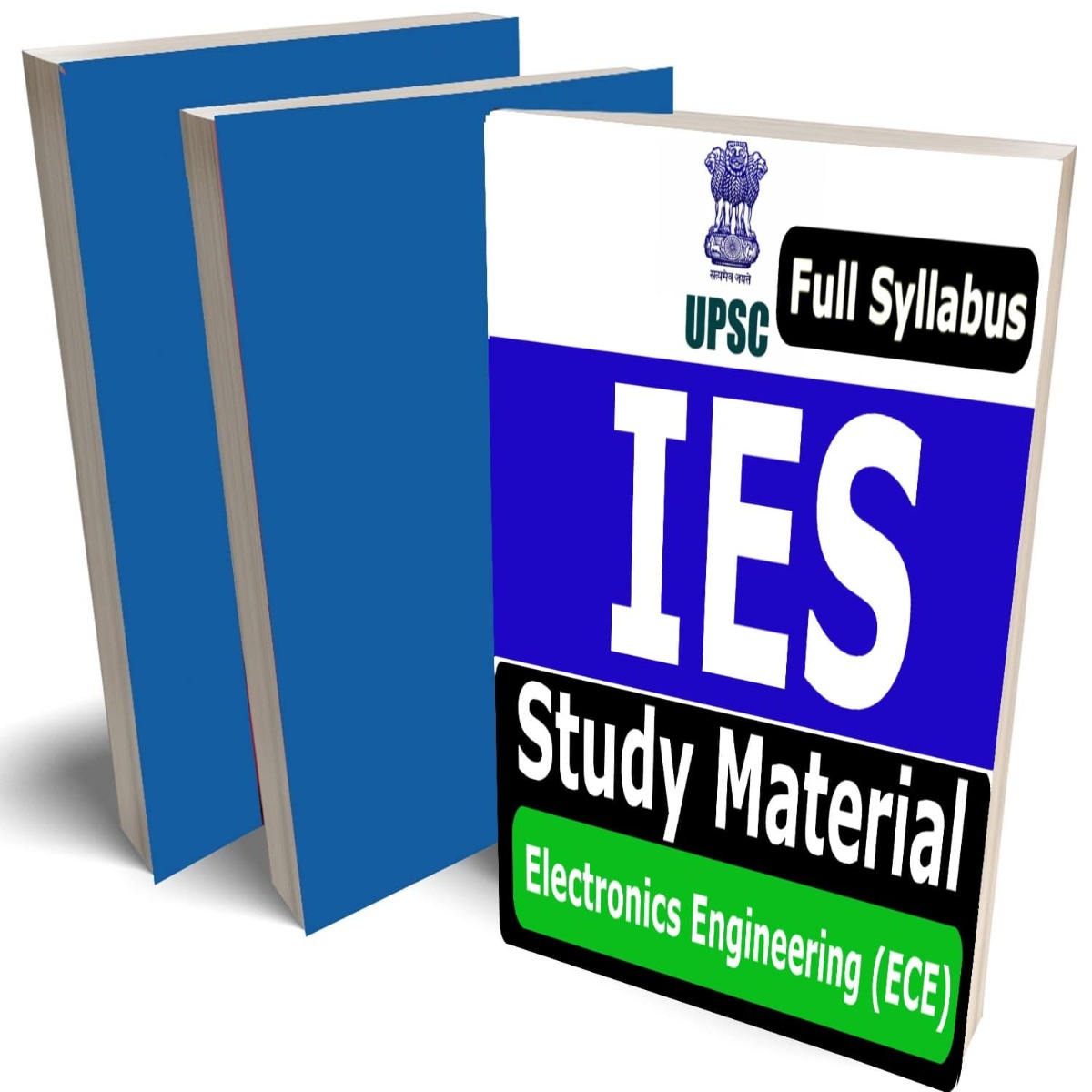
IES Electronics Engineering Study Material (ECE) Lecture Notes (Topic-wise) Buy Online Full Syllabus Covered Books (Study Notes)(GATE, ESE, PSU)
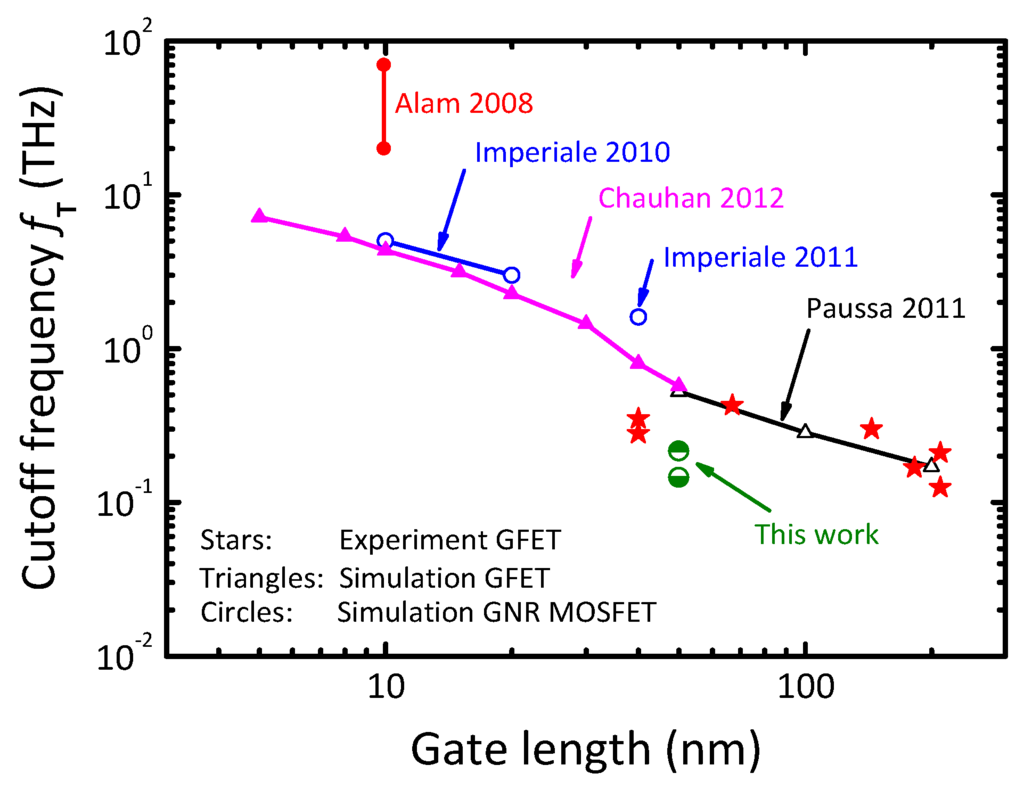
Electronics, Free Full-Text

Solvent-free adhesive ionic elastomer for multifunctional stretchable electronics - ScienceDirect
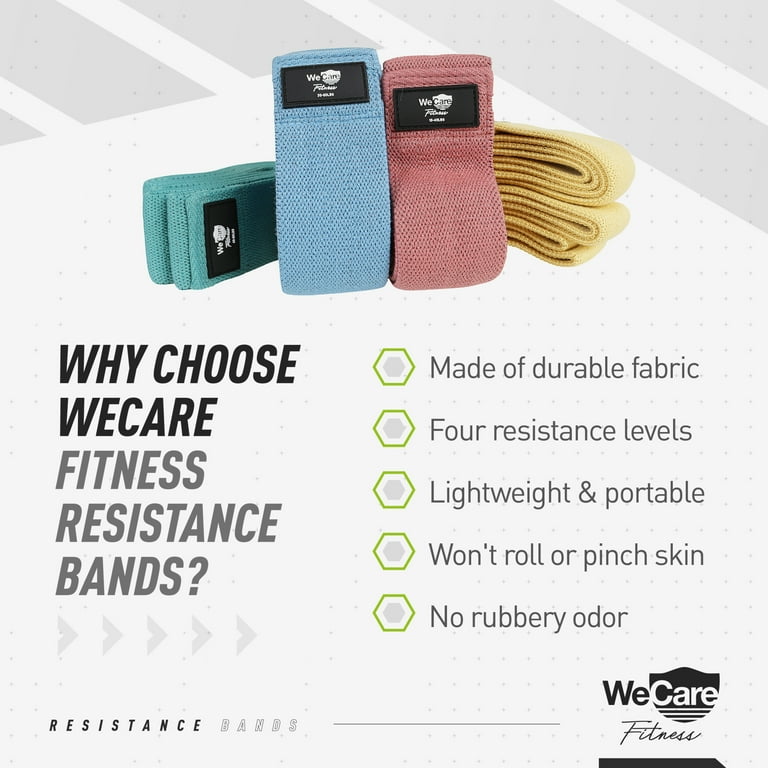
WeCare Latex Free, Full-Body Workout Exercise Bands for Women and Men, 4 Levels of Resistance, Instruction Guide and Mesh Carrying Bag Included, Set Contains 4 Bands

Electronics icon set Royalty Free Vector Image
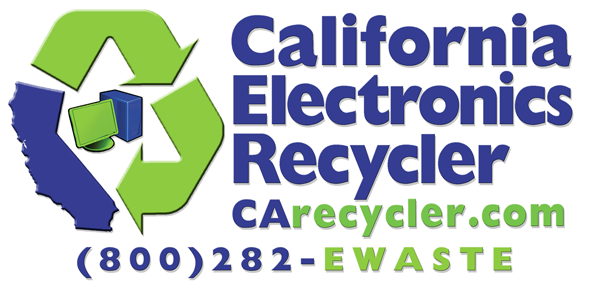
E-Waste Recycling OC, Free Data Destruction
Ali M. Bazzi on LinkedIn: If you are in Connecticut or the Northeast region, please join me and…
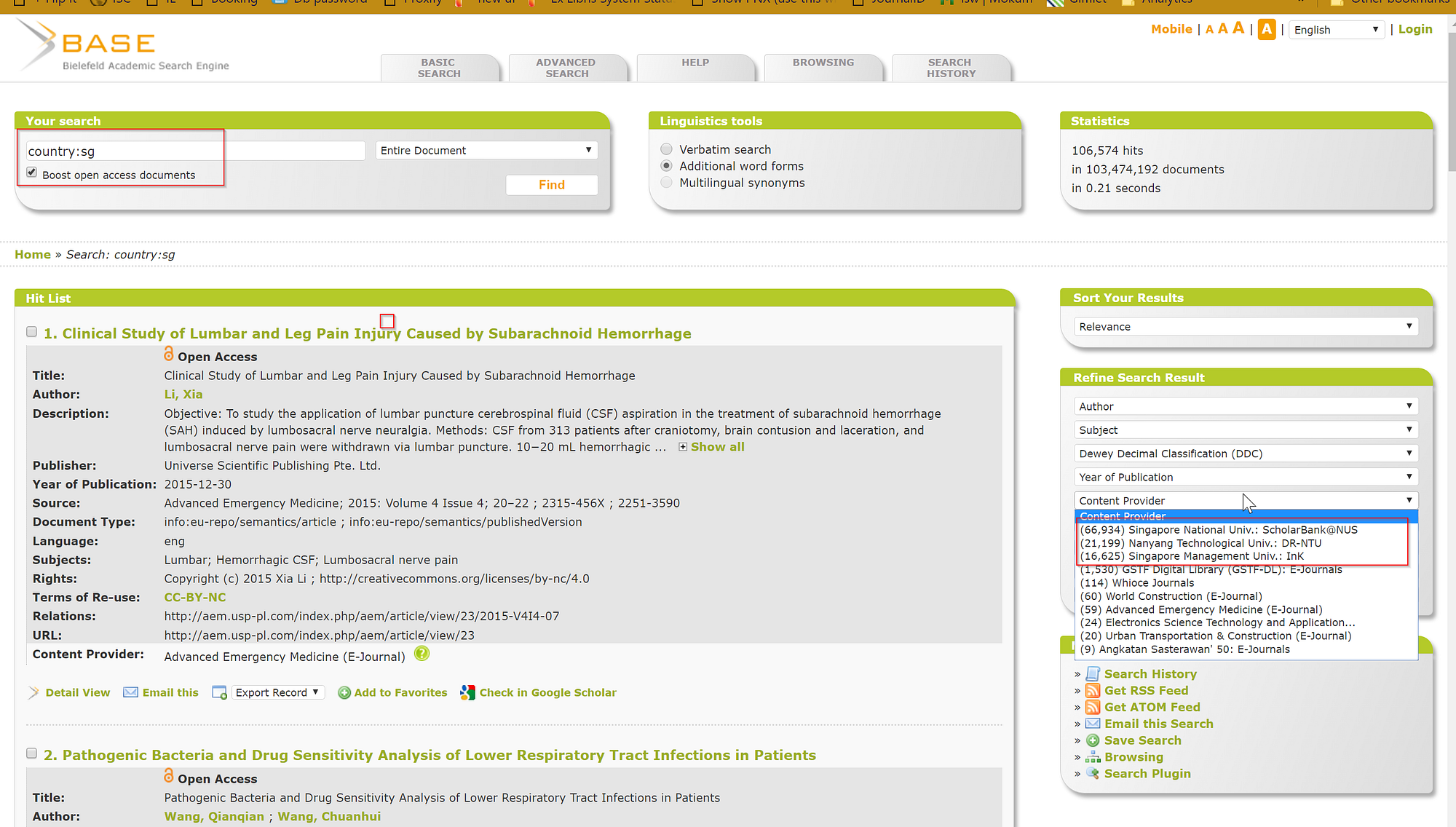
Aaron Tay's Musings about librarianship : The open access aggregators challenge — how well do they identify free full text?

Electronics Terms and Vocabulary (Free Spanish Lessons for Kids)
de
por adulto (o preço varia de acordo com o tamanho do grupo)