Visualizing the gradient descent method
Por um escritor misterioso
Descrição
In the gradient descent method of optimization, a hypothesis function, $h_\boldsymbol{\theta}(x)$, is fitted to a data set, $(x^{(i)}, y^{(i)})$ ($i=1,2,\cdots,m$) by minimizing an associated cost function, $J(\boldsymbol{\theta})$ in terms of the parameters $\boldsymbol\theta = \theta_0, \theta_1, \cdots$. The cost function describes how closely the hypothesis fits the data for a given choice of $\boldsymbol \theta$.
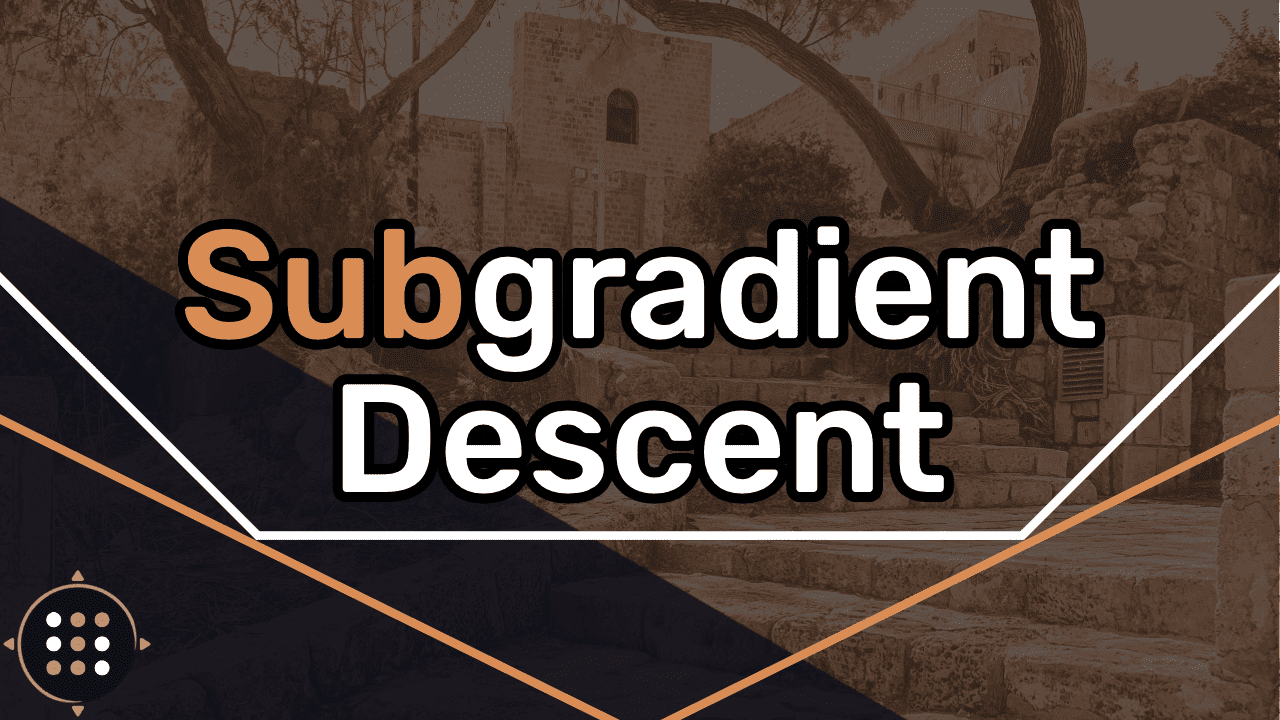
Subgradient Descent Explained, Step by Step
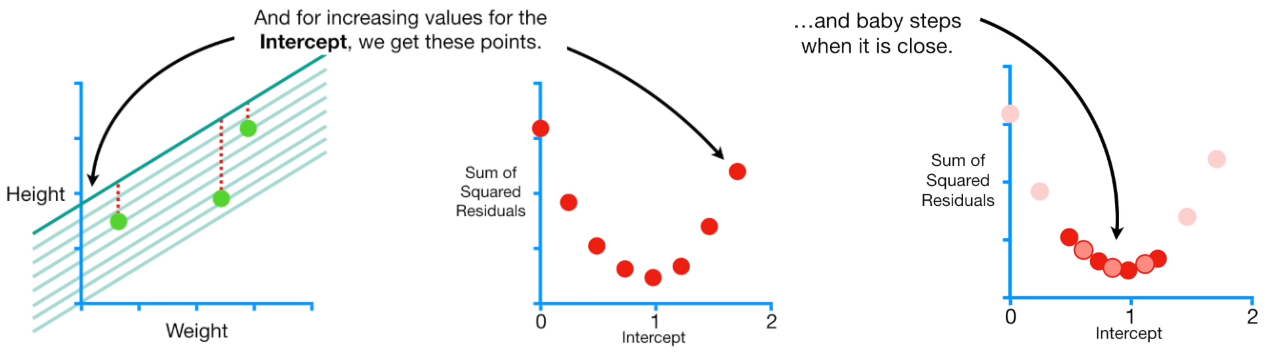
Gradient Descent Step by Step - Andrea Perlato
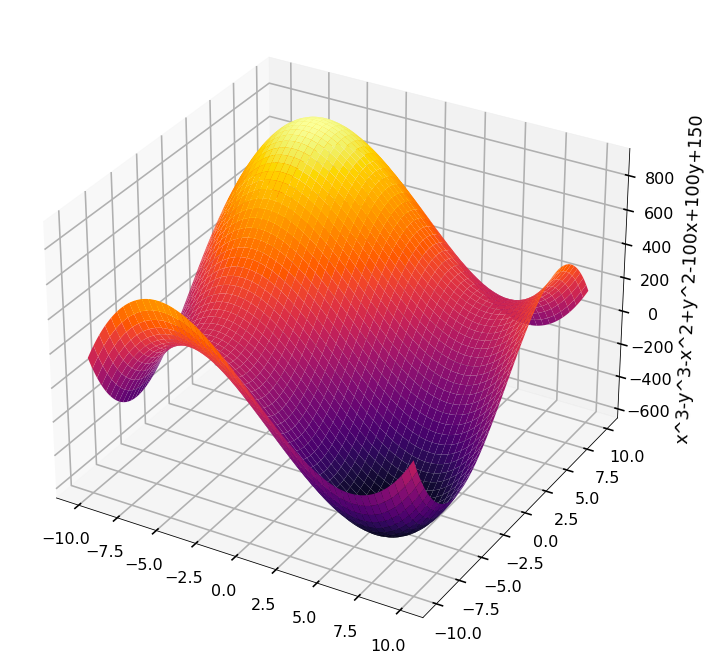
Gradient Descent Visualization - Martin Kondor
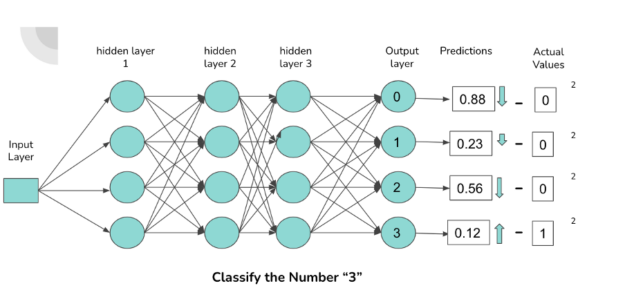
A Data Scientist's Guide to Gradient Descent and Backpropagation Algorithms

How to visualize Gradient Descent using Contour plot in Python
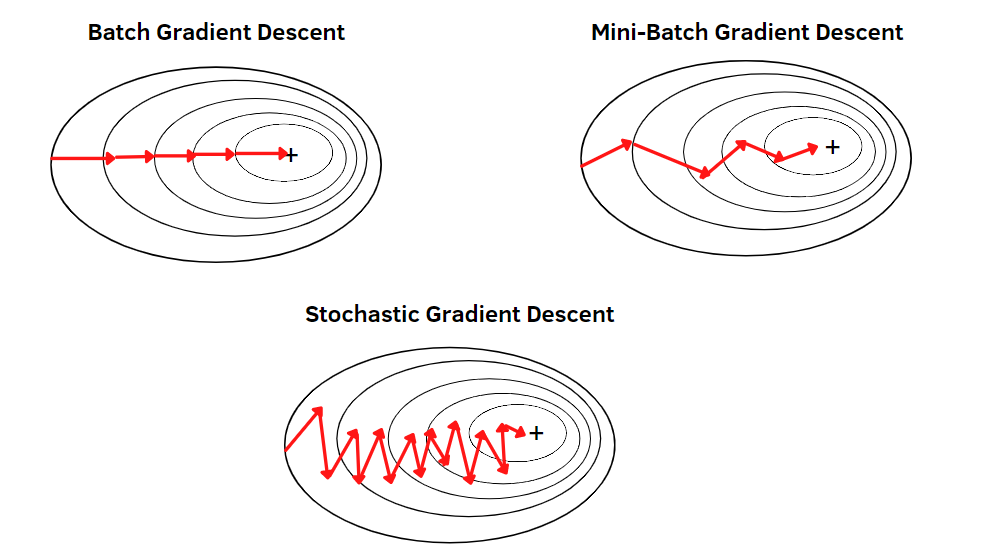
Gradient Descent and its Types - Analytics Vidhya
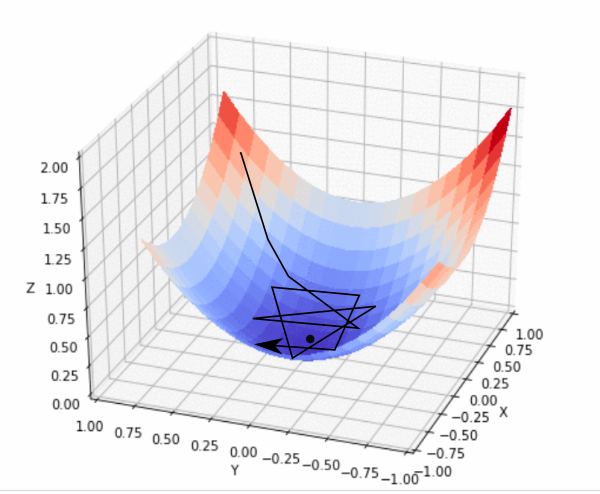
Intro to optimization in deep learning: Gradient Descent
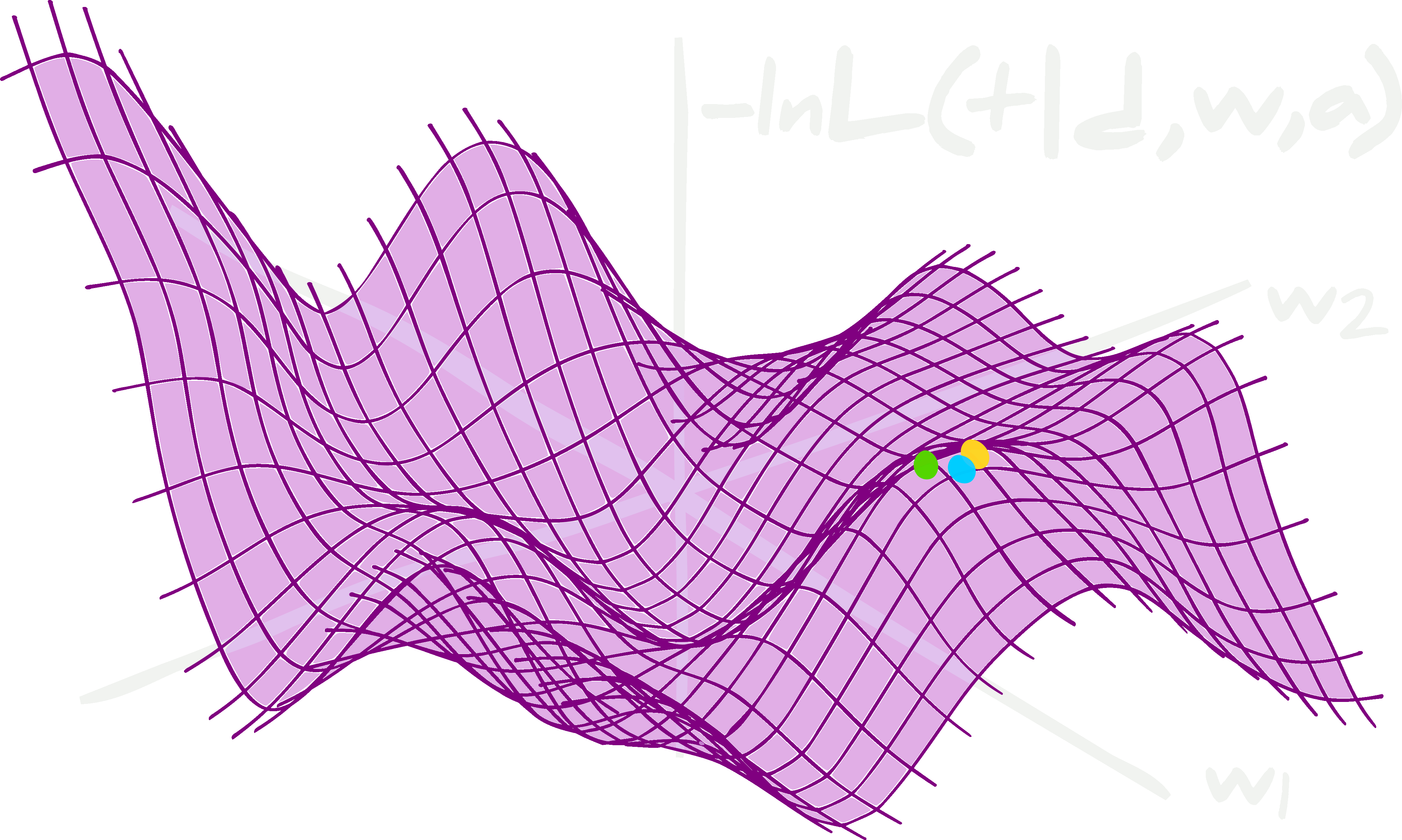
4. A Beginner's Guide to Gradient Descent in Machine Learning, by Yennhi95zz
Visualizing Newton's Method for Optimization II
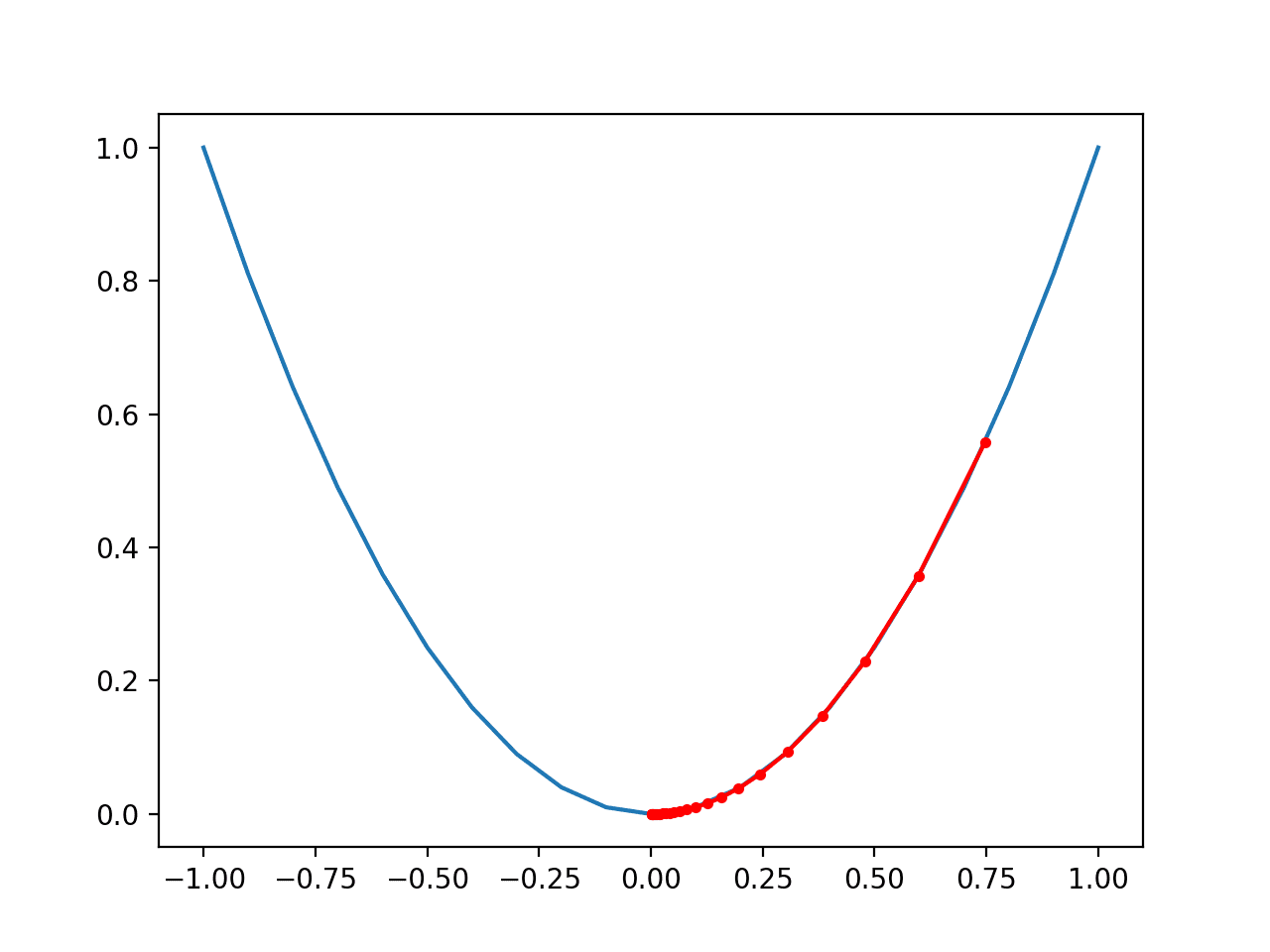
Gradient Descent With Momentum from Scratch
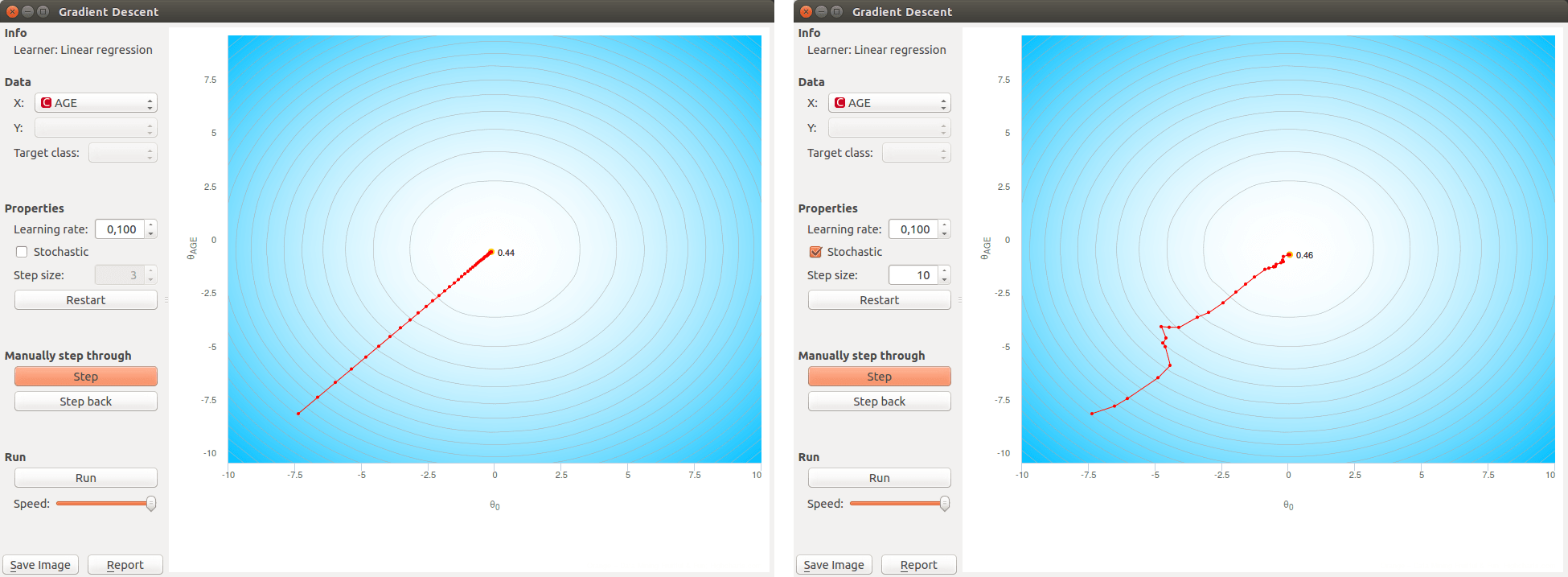
Orange Data Mining - Visualizing Gradient Descent
Gradient-Based Optimizers in Deep Learning - Analytics Vidhya
de
por adulto (o preço varia de acordo com o tamanho do grupo)